In this blog post, I am going to talk about the journey from “zero to one” and all the learnings that came out of it.
We were working on our annual goal-setting exercise and one of the strategic initiatives was to build a “Culture of experimentation“. To give more context, I was working with a company that offers a SaaS product to run A/B tests.
As we were working on our annual initiatives we realized that the biggest bottleneck for experimentation program to be successful is “Culture of experimentation”
To truly help our customers with this shift in the culture we had to do it ourselves to realize the actual challenges that come with it. The initiative started with a “LONG” document about all our learnings from customers that stopped running AB test to research about companies like booking.com, Netflix, Google, etc who had successfully implemented a culture of experimentation.
I will write a separate article talking in-depth about the process but let us straight away talk about the learning when we actually started running experiments!
Learning #1 : Experimentation is beyond A/B testing your website
If you need to build a culture then all your strategic decisions need to undergo experimentation. This learning comes from the pioneers in experimentation – Booking.com, Airbnb, and Amazon among others. Our research on the experimentation principles followed in these companies revealed the depth to which the testing culture was ingrained in them. For instance, at Booking.com, even the job descriptions on their vacancies portal are A/B tested.
For any SaaS company, one of the primary goals is to generate leads, therefore we started experimenting on our website which is when we realized that the conversions on the website depend on multiple factors that we are outside of the scope of the website. For instance what kind of traffic are we generating? Is there a scope of experimenting on marketing channels or can we experiment on content, ads that drive traffic to our website?
Learning #2 : Experimentation culture needs to be built top down
Experimentation requires patience – which is why companies with leadership that promotes instant gratification shy away from investing in experimentation. The return on investment can be spectacular, and the companies we studied in our research proved it, but this is something that would happen over time and requires you to embrace failures. The moment you decide to move away from a couple of ad-hoc experiments to running a program based on a testing roadmap, your difficulties become more cultural in nature than technical or financial. Hence, we realised that we needed Paras, who is the Founder Chairman of VWO, to control the program. His buy-in, and that of the senior leadership, in getting rid of biases and the HiPPO approach to decision making, was most important. With him and other leaders being fully on-board, educating the teams on having the patience to experiment became that much easier. Having leadership buy-in also meant that the company was willing to bear the cost of investing
in the right resources, tools and time.
Building the culture bottom-up is not advisable, as the ROI of experimentation is neither immediate, nor certain.
Learning #3. Identify the right framework, team structure, and goals.
Identifying the right framework, team structure, and having a clear goal are prerequisites for scaling the experimentation program. When you’re starting out on your experimentation journey, you don’t know what’s going to work for you. You can take inspiration from others’ learnings, but with each company being different in its goals and requirements, you cannot overlay someone’s framework on your program. You therefore need to have a group of people experimenting with the experimentation program. So we were clear on the team structure we wanted to follow – we built a core team that would focus on experimentation, derive learnings, and become experimentation evangelists for everyone else in the company.
Our core team was pretty small initially. Having used VWO for a long time , I acted as a product expert and a program manager. We also included a dedicated data analyst in the team along with a shared engineering resource (who was later turned into a dedicated resource as we started running complex tests).
As a POC project, this core team of three worked with the demand gen marketing team – so test owners were included from paid marketing as well as organic search. These owners were all responsible for research and ideation.
Our research also revealed that we would fail if our experimentation was aimless, that is, if the experimentation goals were not linked to business KPIs. It had to be as specific as possible and align with the business strategy. One of our business goals is to move upmarket. So we put considerable thought into how we could tie the business strategy with experimentation and the goal we then identified was to increase leads for mid-market and enterprise segments
Our experimentation team’s focus area was going to be marketing, so we needed a marketing goal but we could not work with a vague goal like generating leads
Learning #4. Don’t let operational issues become a hindrance
Whether it’s setting aside a separate budget for running the experimentation program, investing in tools and resources, dedicating time, or anything else – we learned that operational issues should be taken care of before they hinder the success of your program. We didn’t want our core experimentation group to fret over how to find the data for experiments, or which tools to use for that. At VWO, it’s important for us that the team focuses on ideation. So for instance, even before we started the program, we created an experimentation dashboard in Tableau. We also integrated Google Analytics (GA) in VWO, so that we could deep dive into the performance of our experiments in GA. We had our goals in place so we identified the data points we needed and the tools that would provide us this data, along with the tools that we needed to procure.
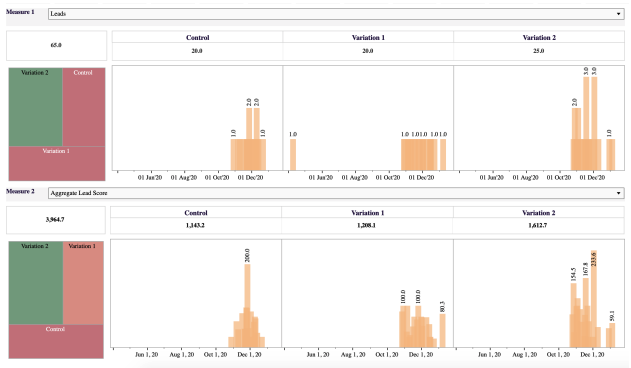
Learning #5. Build culture first and then scale your program.
One important mantra for experimentation that we’ve learned is to start small, and start early. At VWO, we may not be able to run thousands of experiments simultaneously today – but we will get there eventually. What is important is that we started on this as early as possible, and though we started with a small team, we are now in the second phase of our program where representatives from multiple teams are using experimentation as a basis for their decisions.
If you’re a startup, you’re going to take the most important decisions in your early days – so investing in experimentation and building the right culture for it becomes more important, and would prove more beneficial as well.
Our approach to building culture incorporates four major steps:
1. Being data driven | – Making data easily accessible to everyone – Insisting that data eats opinions for breakfast, anyday |
2. Working with the right set of tools. | – Mastering the use of VWO to gain insights and conduct experiments – Identifying any gaps in our product and passing on feedback – Procuring any tools that are needed for access to important data points |
3. Evangelizing both the wins and the failures | – Building curiosity among teams about the tests being conducted through discussions, games, polls, etc. – Sharing the results of experiments with the insights (learnings) highlighted |
4. Defining the experiment protocol as clearly as possible | – Building and evolving checklists – Documenting the workflow – Identifying experiment owners |
Learning #6. The experimentation team cannot work in a silo.
Irrespective of the team structure you opt for, cross-team communication is mandatory. We realised that the experimentation team’s goal of increasing leads for mid-market and enterprise segments could not be achieved with the isolated efforts of this group. A framework had to be put in place in the rest of the organization to support this goal too.
We did not want to risk being in a situation where, for example, an experiment is being run on the website but the paid marketing team is not aware of the experiment team’s goals and is hence not focusing on acquiring enterprise traffic.
Therefore we did the below:
- Communicated the goals to the entire organization
- Aligned the outbound sales team with the goals so they could focus on the enterprise
market - Built a section catering to the needs of the mid-enterprise audience on the website ( this
was passed through the lens of experimentation)
As the program scales and more teams are involved in experimentation, their goals can also be aligned accordingly. So at VWO, while the core team’s goal remains the same, they communicate their overarching experimentation framework to other teams. Therefore, say when the product team is on-board with experimentation, their goals could be aligned accordingly. (For them the goal could be product adoption for mid-market and enterprise, but the philosophy of deducing the goal from business KPIs remains intact).
Learning #7. Checklists ensure that there are minimum quality issues.
While we’ve always said that VWO makes A/B testing easy, for someone new to experimentation there can be fears associated with making changes – what if something goes wrong on the website? That is where a checklist plays a significant role.
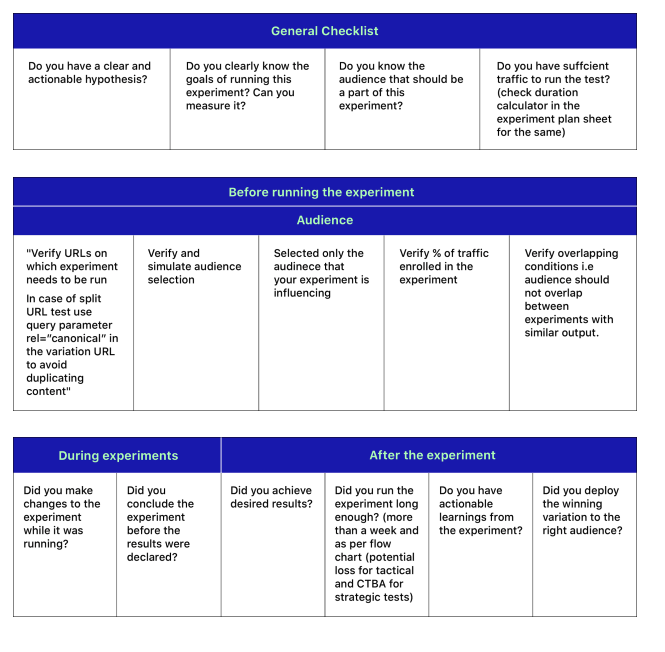
checklist template here.
Checklists make sure that the obvious steps in experimentation are not missed out, and instill a sense of confidence in the team.
Our checklist was divided into three parts: before experimentation, during experimentation, and post experimentation
Something seemingly obvious like – What is your goal for this experiment? Or, do you have an actionable hypothesis? – can be overlooked by a test owner who’s just starting out, and a checklist wipes out the possibility of that. This goes a long way in easing the hesitations of new experiment owners.
The core experimentation team also evolved the checklist based on their experience and made it as tactical as possible. So a checkpoint that previously said – Have you performed audience segmentation? – was later refined to – Have you identified the audience you want to influence with this test and segmented accordingly?
Learning #8. Make teams comfortable with the idea of experimentation
When you’re going from zero to one in your program, making the team comfortable with the idea of experimentation is crucial. The process of ideation gets better, with an improvement in both quality and quantity, if you’re able to build the right cadence in testing.
Don’t get me wrong here – prioritization of hypotheses is a crucial step in experimentation. But when you’re starting out, you might not have a backlog of hundred ideas. You would most likely have a couple of ideas, and your priority at this stage should be to get the basics right. Your aim should be to test out all the ideas you have, run multiple experiments in parallel when needed, and become well-equipped to execute experiments.
Our ultimate aim was to build culture, so we wanted to optimize for learnings and velocity, and not so much for results
As we went from zero to one, we wanted team members to get comfortable with testing, getting positive results that moved our metric was like a happy byproduct of that. We wanted to work on the philosophy that no idea was wrong until tested, and only the experiment results would kill our biases. This was more important than counting the wins.
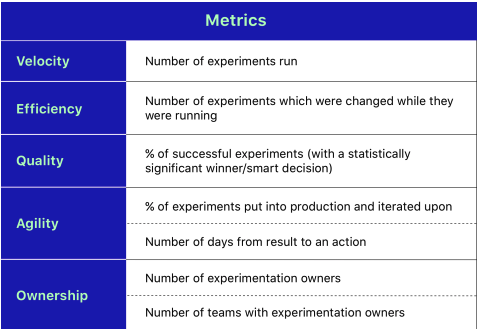
Learning #9. Feed your operational learnings back into experiments.
You’re prone to errors when you’re in the zero to one phase. Selecting the right goals for every test, defining the correct audience, and other such nuances of experimenting can be learnt only as you test more and more. It’s important to be conscious of these mistakes though, and feed every learning back into your testing roadmap. For instance, if you’re optimizing a free trial modal by removing a particular field, you need to include the audience who are opening the modal. If you include visitors who are not interacting with the modal, they are going to skew your numbers. (Which is why a checklist is important and having tactical checkpoints which don’t leave space for ambiguity is crucial.) Taking learnings from the modal example, we ensured that when we were running an A/B test on the footer section of our blog, we only targeted the audience that was scrolling close to the footer. Since we were measuring the efficiency of our tests, and tracking our mistakes, we could identify where we needed to improve and how we could do so.

Learning #10. Keep an eye on your guard-rail metrics at all times.
Many companies have their primary and secondary metrics in place while experimenting, but fail to identify and measure guard-rail metrics. Your primary metrics decide whether an experiment is worthy of deployment – these could be the number of free trials or the number of demos requested. Secondary metrics support the primary metric – like engagement, scroll rate, etc. While it is imperative to measure your primary and secondary metrics, it is equally
important to check that if these are moving, what the impact on the guard-rail metrics is. Without this, chances of you going downhill are high.
For instance, say your primary metric is the number of free trials and your guard metric is lead score. So as your free trials go up, your lead score should not go down either. If it does, you know that’s a red flag and it will stop you from celebrating prematurely. In one of our experiments at VWO, we were testing the headline on our homepage. Our primary goal was to check MQLs generated.
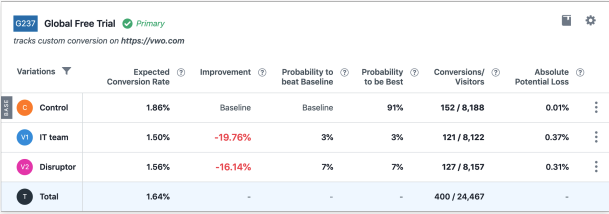
The result above shows that the control is generating more leads than the other two variations. The probability of it being the best among the three was greater than 90% and we could have easily made a decision in favor of control. However, one of our guardrail metrics was average lead score. Based on our formula to calculate the quality of leads, we checked for an average lead score and found, to our surprise, that it was the lowest for the control. This made us rethink our decision.
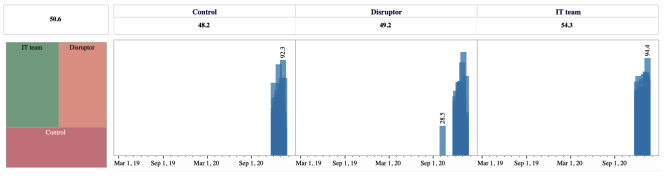
I hope you liked the post! Do reach out to me if you have any thoughts or questions!